Alright, let’s dive into this “man u xg” thing. So, basically, I was messing around trying to get my head around expected goals (xG) in football, specifically looking at Manchester United’s data. Sounds nerdy, right? Well, it is.
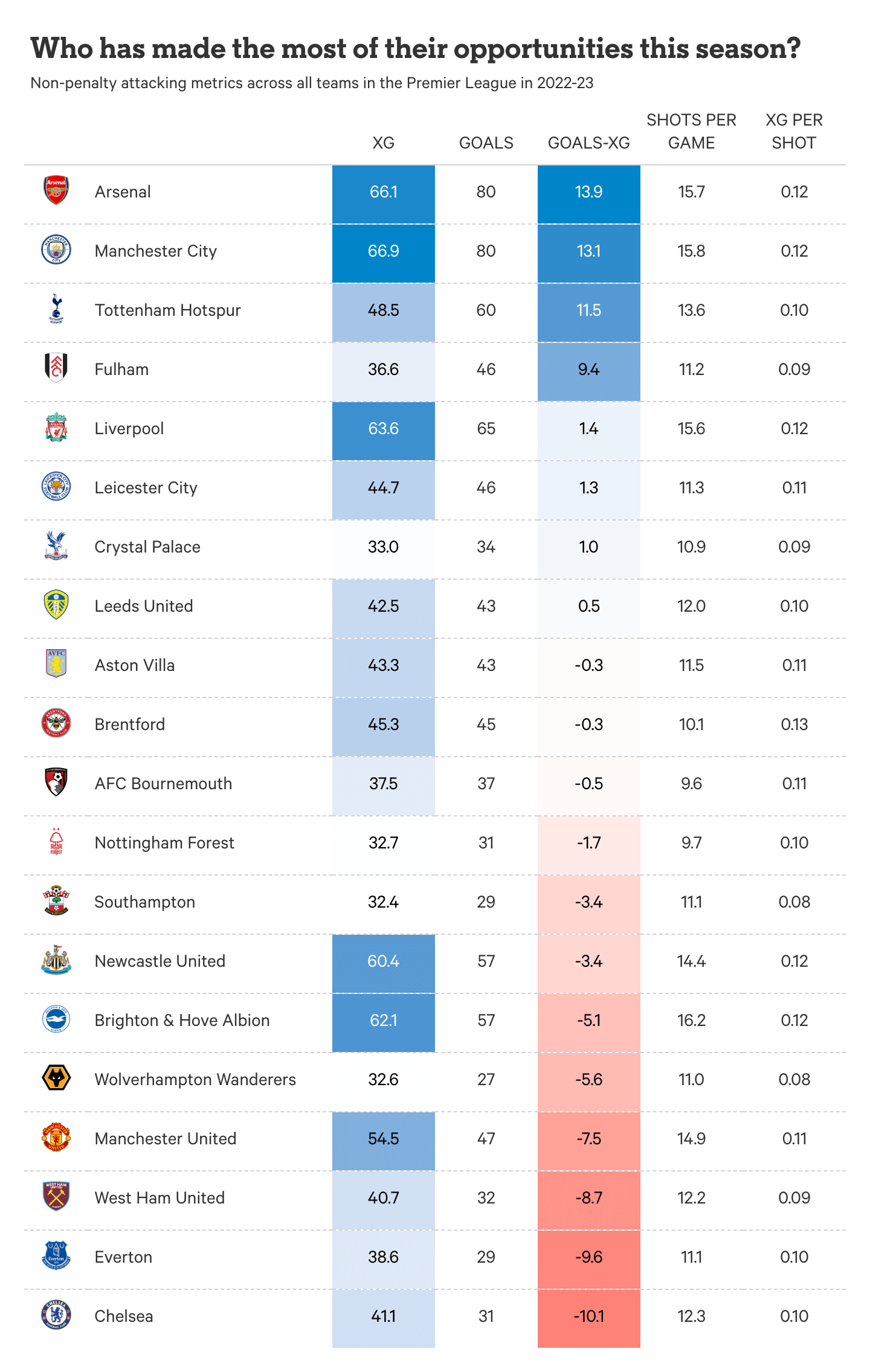
First off, I grabbed some data. I found a website – can’t remember which one now, there are tons out there – with game-by-game data including xG for each team. I copy-pasted it into a spreadsheet. Yeah, I know, super high-tech.
Then came the fun part – trying to actually make sense of the numbers. I was trying to see if Man U was consistently over or underperforming their xG. Like, were they scoring more goals than their xG suggested they should, or were they choking and missing easy chances?
Here’s what I did step-by-step:
- Calculated xG difference: For each game, I subtracted Man U’s xG from their actual goals scored. This gave me a positive number if they overperformed, and a negative number if they underperformed.
- Averaged it out: I calculated the average xG difference over a whole season (or part of a season, whatever data I had). This gave me a sense of their overall performance relative to their xG.
- Looked at trends: I plotted the xG difference over time to see if there were any patterns. Were they getting better or worse as the season progressed? Did certain players seem to have a bigger impact on over/underperformance?
What did I find? Well, honestly, nothing earth-shattering. Some seasons they were slightly above their xG, some slightly below. No massive revelations that would explain all of Man U’s woes (or triumphs). It was more just a way to visualize their performance and get a slightly more nuanced view than just looking at the raw scorelines.
I tried to break it down further, looking at home vs. away games, games against top teams vs. games against lower-ranked teams, stuff like that. Again, nothing super conclusive, but it was interesting to see how those factors might influence the numbers.
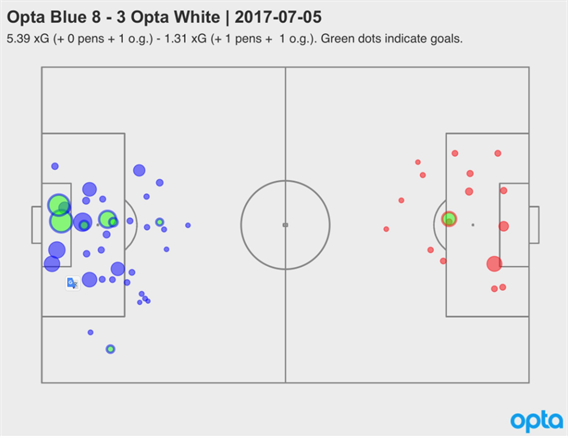
The trickiest part was figuring out why they were over or underperforming. Was it luck? Goalkeeping? Finishing ability? Hard to say for sure just from the xG data alone. You really need to watch the games and dig into the specific chances they were creating or missing.
So, in the end, “man u xg” was more of a learning exercise than a groundbreaking analysis. I got a better understanding of how xG works, how to manipulate the data, and how to think about football performance in a slightly more analytical way. Plus, it was a fun way to waste an afternoon.
Future stuff to try: I’d like to incorporate more data, like shot locations, pass maps, and defensive pressure metrics. Maybe even try to build my own xG model. Who knows, maybe one day I’ll become a football data guru. Probably not, but hey, a guy can dream!